Ficha Técnica de los autores de los seminarios (2020 - 2021)
Lavinia Amorosi
CV: Lavinia Amorosi is Researcher in Operations Research at the Department of Statistical Sciences of Sapienza, University of Rome. She received the Ph.D in Operational Research from Sapienza University of Rome in 2018. Her research area is mainly on combinatorial optimization, with particular interest in network optimization and multi-objective programming, with application to real network problems in telecommunication, energy and transportation areas.
Abstract: Complexity of problems deriving from real applications is leading to an increasing attention and adoption of multicriteria optimization in several sectors. One of these is the one of energy where new hybrid configurations also involving renewable energy sources targeting self-sufficiency are currently studied. In this talk I present a novel mathematical formulation to solve the problem of optimally sizing and managing battery energy storage for solar photovoltaic (PV) system integration of a multi-apartment building. The aim is the maximization of the collective self-consumption maintaining the control over time of the energy sold and bought and the monitoring of batteries state while it ranges from the minimum to the maximum levels. This is obtained through a new mathematical programming model with three different objective functions which can be simultaneously tuned to find problem Pareto optimal solutions. The computational results on detailed scenarios with real data verify the model adequacy to the real problem and give a measure of saving of bought and sold energy.
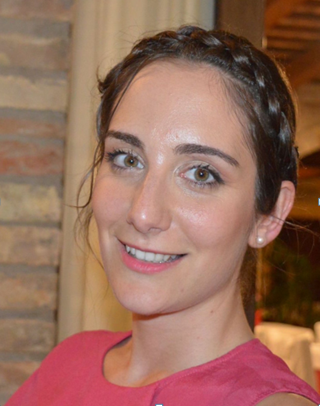
Marina Leal
CV: Marina studied Mathematics at the University of Alicante and obtained a Master in Statistics and Operations Research at the University of Murcia. She received her PhD in May 2019 from the University of Sevilla. She served as a postdoctoral researcher in the research training group “Algorithmic Optimization” at Trier University. Currently, she is working as a Professor at the Miguel Hernández University. Her main research interests comprise mixed-integer linear and nonlinear programming problems in different fields as bilevel optimization or robust optimization and diverse applications such as location and transportation theory, portfolio optimization, or energy problems.
Abstract: J.F. Benders introduced in 1962 a decomposition technique, currently known as Benders Decomposition, to solve problems with complicating variables. Since then, and especially in recent years, this technique has been extended and applied to a wide variety of optimization problems such as bilevel, robust, multiobjective, or stochastic problems, in many different applications such as location, transportation, finance, or energy. It allows to exploit the structure of the problem and to decentralize the computation, which results in the possibility of solving big problems. In this talk, we will go over the classical version of this Benders Decomposition technique, its generalizations, extensions, and improvements through concrete optimization applications.
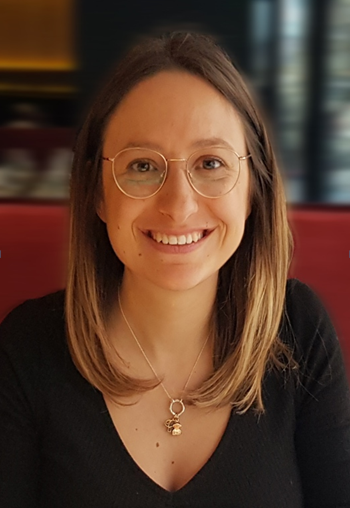
Víctor Blanco
CV:Graduate in Mathematics at the Universidad de Granada (UGR) and PhD in Operations Research at the Universidad de Sevilla. Since 2009, he is a researcher at the UGR. Currently, as associate professor at the IEMath-GR and the Dpt. of Quantitative Methods for Economics of Business. His research interests are in different topics related to mathematical programming, both theoretical and applied, with special interests in the applications of optimization tools in (discrete and continuous) facility and hub location, data science and routing problems.
Abstract: In this talk we introduce a new version of the maximal covering location problem in a continuous framework, in which the services to be located are required to be interconnected by means of a graph structure in which two facilities are allowed to be linked if a given distance is not exceed. These ‘linking requirements» are useful when finding the location of telecommunication servers, that in case of being adequately connected, are able to provide a more accurate service by sharing capacity with other servers or providing service to customers allocated to another facility in case of failure. We provide a general mathematical programming framework for the problem and different resolution strategies. We will derive some geometrical properties of the problem that allow us to project the continuous variables out avoiding the nonlinear constraints, resulting in an equivalent pure integer programming formulation. Since the number of constraints in the integer programming formulation is large and the constraints are, in general, difficult to handle, we propose two branch-&-cut approaches that avoid the complete enumeration of the constraints resulting in more efficient procedures. We will show the results of our computational experience comparing the performance of the different approaches. This is a joint work with Ricardo Gazquez (PhD student at UGR).
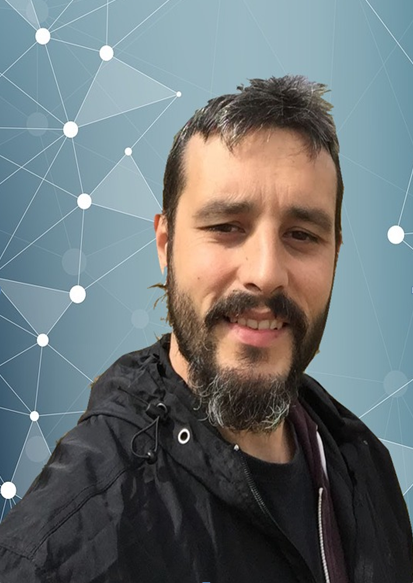
Francisco Saldanha
CV: Francisco Saldanha da Gama is professor of Operations Research in the Department of Statistics and Operations Research at the Faculty of Science, University of Lisbon, where he received his PhD in 2002. He has taught undergraduate and post-graduate courses focusing on Operations Research, Mathematical Programming, Discrete Optimization, Stochastic Optimization, and Logistics. His research interests include stochastic mixed-integer optimization, location theory and project scheduling.
Abstract: In stochastic facility location it is often assumed that there is some CDF representing the underlying random vector, which is known beforehand. Unfortunately this may not be the case since such distribution may also be uncertain. This seminar focuses on this aspect. In particular, the use of adaptative distributionally-robust optimization in the context of capacitated facility location problems is discussed. Different modeling aspects and algorithmic methodologies are covered.
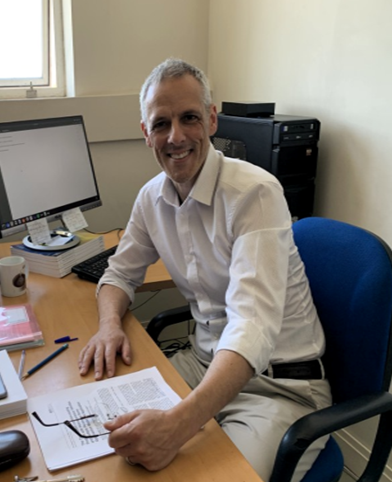
J.F. Monge
CV: Juan Francisco Monge es Licenciado en Matemáticas por la Universidad de Valencia y Doctor en Estadística e Investigación Operativa por la Universidad Miguel Hernández de Elche. Su principales areas de investigación se han centrado en la optimización combinatoria y la optimización bajo incertidumbre, habiendo publicado más de 30 artículos de investigación. Actualmente dirige el grupo de investigación en Gestión de Recursos dentro del Intituto de Investigación Operativa de la Universidad Miguel Hernández de Elche.
Abstract: Dado un ranking de elementos, elementos que pertenecen a diferentes conjuntos, este ranking no implica generalmente un orden total entre los conjuntos. En este trabajo se presentará el problema de ordenamiento lineal de conjuntos y elementos basado en el problema de optimización combinatoria, Linear Ordering Problem (LOP). Se introducirá el coeficiente de concordancia para medir el desorden de los elementos, permitiendo obtener el orden de conjuntos más cercano a un orden de elementos dado. Para la obtención de la medida de concordancia se utilizará la distancia de Kendall, lo que permitirá también presentar el coeficiente de concordancia como generación al coeficiente de correlación de Kendall, permitiendo así medir la relación existente en dos o más muestras. De esta forma, el coeficiente de concordancia permite definir una prueba no paramétrica alternativa a la prueba Kruskal-Wallis. Se presentarán algunos resultados teóricos que permiten establecer la robustez del ordenamiento propuesto, así como algunas aplicaciones: ordenación de países a partir del orden de los colegios (informe PISA), ordenación de tenistas a partir del orden de estos en las distintas estadísticas de juego, ordenación de las características más importantes para el juego de un tenista a partir del ranking ATP de jugadores.
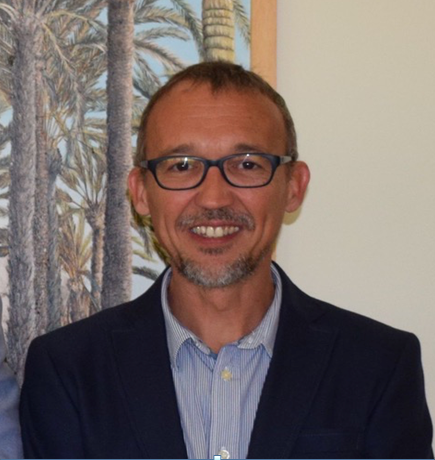
A. Marín
CV: Alfredo Marín es licenciado en Matemáticas por la Universidad de Granada y doctor por la de Murcia, en cuyo Departamento de E. e Investigación Operativa trabaja actualmente como catedrático. Sus trabajos se inscriben en el campo de la Optimización Discreta, con especial atención a los problemas de Localización. Durante este semestre lleva a cabo una estancia de investigación en el IMUS.
Abstract: Los problemas de «rank pricing» aparecen al tratar de determinar los precios idóneos para los productos que una compañía va a poner en el mercado, con el conocimiento previo de las posibilidades económicas y deseos de los potenciales compradores. Aunque en origen son problemas binivel, es sencillo formularlos como problemas de un nivel, no lineales, en variables discretas. La linealización de estas formulaciones nos da una excusa para adentarnos algo en el mundo de la optimización discreta eficiente.
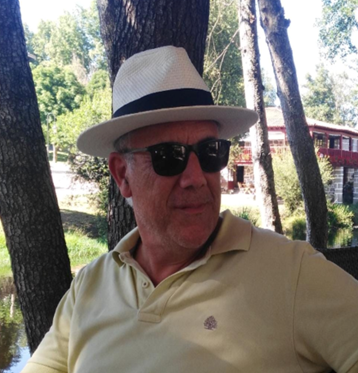
A. Corberán
CV: Ángel Corberán is Professor of Statistics and Operations Research at the Faculty of Mathematics of the University of Valencia (Spain). He has published over 70 articles on combinatorial optimization and is associate editor of Computational Optimization and Applications and TOP, and a member of the Editorial Board of Computers & Operations Research, EURO Journal on Transportation and Logistics, and EURO Journal on Computational Optimization. His research interests focus on the study and solution of combinatorial optimization problems, mainly in the areas of routing and location.
Abstract: Given a network (roads, power lines, pipelines, etc.) and a set of lines that are required to be covered (serviced), each one with an associated cost, arc routing problems (ARPs) consist of finding a tour (closed walk starting and ending at the depot), or a set of tours, covering all the required lines with minimum total cost. In this talk we discuss the ARPs where the vehicles used to cover the required lines of the network are drones (Drone ARPs) and we comment their relation with well-known postman ARPs ([1]). Applications for Drone ARPs include traffic monitoring and infrastructure inspection. Unlike (ground) vehicles in classical ARPs, drones can travel directly between any two points without following the edges of the network. Thus, a drone route may service only part of an edge, with multiple routes being used to cover the entire edge. Therefore, Drone ARPs are continuous optimization problems in which the shape of the lines to be serviced must be taken into account. To deal with this feature, we discretize the problems by approximating each curve by a polygonal chain and allowing the drones to enter and leave each curve only at points of the polygonal chain. If the autonomy of the vehicles is unlimited, the resulting problem is a rural postman problem (RPP), otherwise we have the length constrained K-drones RPP, where a fleet of drones has to service a set of edges of a network ([2]). Drone routes have to start and end at a given vertex and, since the autonomy of the drones is restricted, the length of their routes is limited by a maximum distance. Furthermore, if, as in some applications, drones also have to deliver some goods to a set of customers, represented as vertices in the network, we have the multi-purpose K-drones general routing problem, which combines the service to nodes and arcs of the network under capacity and length constraints. In this talk we present some results on all these problems.
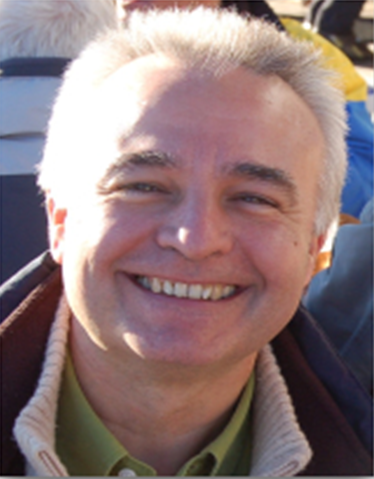
M. A. Pozo
CV: Miguel Pozo es Licenciado en Matemáticas por la Universidad de Sevilla donde obtiene posteriormente el Máster Propio en Estadística Pública. En julio del 2015 defendió la tesis doctoral titulada «Mathematical Models for the Design and Planning of Transportation on Demand in Urban Logistics Networks» dentro del Programa Oficial de Doctorado en Matemáticas. Tras haber realizado estancias de investigación en universidades como las de Gotinga, Montreal, Bruselas y Cádiz, actualmente es profesor en el Departamento de Estadística e Investigación Operativa de la Universidad de Sevilla. Desde el inicio de su actividad investigadora se ha encargado del desarrollo de la línea de investigación «Optimización discreta» y su aplicación tanto a problemas de localización como a redes de transporte. Asimismo, su investigación aborda diferentes problemas de optimización multiobjetivo para el diseño de redes.
Abstract: Let G be a given graph whose edge set is partitioned into a set of red edges and a set of blue edges, and assume that red edges are weighted and contain a spanning tree of G. Then, the Stackelberg Minimum Spanning Tree Game (StackMST) is that of pricing the blue edges in such a way that the total profit of the blue edges selected in a minimum spanning tree of the resulting graph is maximized. A recent contribution (see Labbé, M., Pozo, M.A. and Puerto, J. 2020, Computational comparisons of different formulations for the Stackelberg minimum spanning tree game. Intl. Trans. in Op. Res. doi:10.1111/itor.12680) presents a catalog of new mathematical programming formulations for the StackMST (based on the properties of the minimum spanning tree problem and the bilevel optimization paradigm). The most successful formulation uses an incomplete representation of the spanning tree polytope that requires to add cut-set inequalities to be included dynamically in a Branch-and-Cut algorithm. Besides, it is known that alternative StackMST formulations that do not require the strong duality property exist. Based on this, we present in this paper different advances in the StackMST supported by new formulations and solution methods. In particular, we impose minimum cost optimality constraints to improved path-based STP formulations. We establish a theoretical and empirical comparison between these new formulations that are able to solve random instances of 20 to 70 nodes. We also test our models on instances in the literature, outperforming previous results.
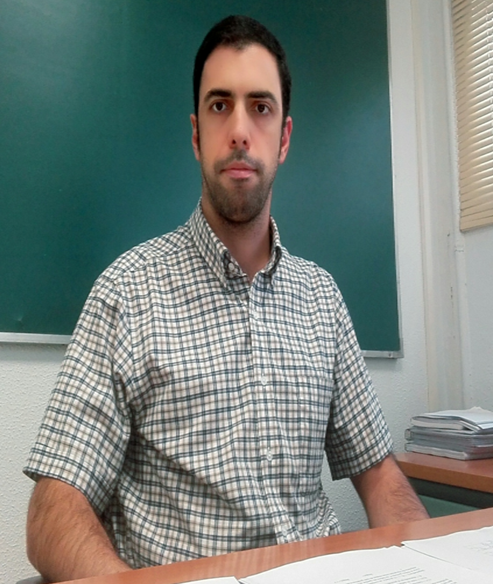
D. Ponce
CV: Diego Ponce has been researching since 2012 different strategies to solve Combinatorial Optimization Problems in the field of location, networks, voting or data analysis. In 2016, he obtained a jointly supervised PhD from Universidad de Sevilla and Université Libre de Bruxelles. After that, he has worked as a postdoctoral fellow or a lecturer in Universidad de Sevilla, Concordia University, Universidad de Granada and currently he is working in Universidad de Zaragoza.
Abstract: The Graph-Connected Clique-Partitioning Problem (GCCP) is a clustering optimization model in which units are characterized by both individual and relational data. This problem, introduced by Benati et al. (2017) under the name of Connected Partitioning Problem, shows that the combination of the two data types improves the clustering quality in comparison with other methodologies. In this talk, after the introduction of the GCCP and its first formulations, we will provide a new Integer Linear Programming (ILP) formulation, that solves the problem using an exponential number of variables.
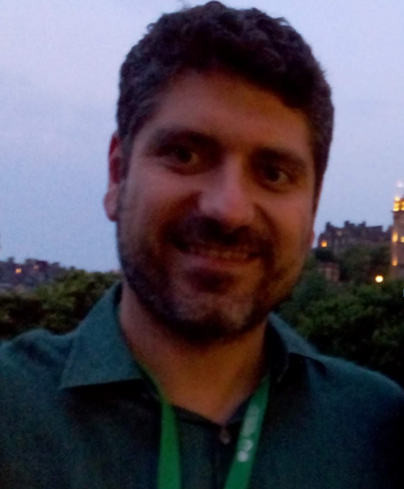
J. J. Peiró
CV: Juanjo Peiró is assistant professor at the Department of Statistics and Operations Research at Universitat de València (Spain). His teaching focuses on Mathematical Programming and Statistics for life and social sciences, and his research interests involve Operations Research in general, and Combinatorial Optimization in particular. His teaching focuses on Mathematical Programming and Statistics for life and social sciences, and his research interests involve Operations Research in general, and Combinatorial Optimization in particular. He enjoys working on optimization problems with discrete settings, especially those related to network design, routing, facility location, and graphs. He likes developing and applying integer and mixed-integer programming techniques, as well as heuristic and metaheuristic algorithms, to tackle deterministic and stochastic versions of such problems.
Abstract: We study the capacitated dispersion problem in which, given a set V of nodes (facilities), a positive capacity ci for each node i, a nonnegative distance dij between any pair of distinct nodes i and j and a positive demand B to cover, we would like to find a subset of nodes V’ such that Σ{i∈V’}ci ≥ B and min{i,j∈V’: i≠j} dij is maximized. For this purpose, we present several integer programming formulations, propose families of valid inequalities to strengthen them and methods to solve the related separation problems when the valid inequalities are exponential in number. The formulations we present have some variables in common, so we try to compare their relaxation bounds. Finally, we conduct a computational study to see the performances of our proposals.
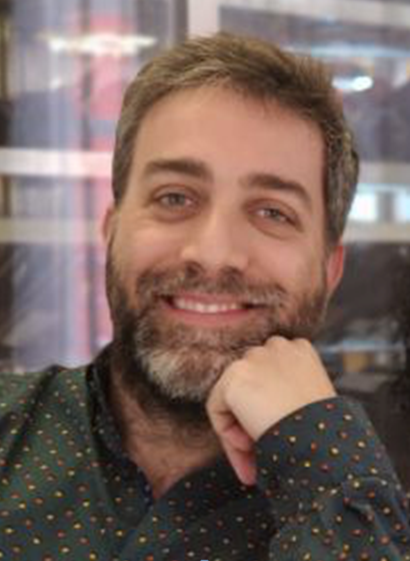
Rubén Ruiz
CV: RUBEN RUIZ is a Full Professor of Statistics and Operations Research at the Polytechnic University of Valencia, Spain. He is co-author of more than 80 papers in International Journals and has participated in presentations of more than 150 papers in national and international conferences. He is editor of the Elsevier journal Operations Research Perspectives (ORP) and co-editor of the JCR-listed journal European Journal of Industrial Engineering (EJIE). He is also associate editor of other important journals like TOP and Applied Mathematics and Computation as well as a member of the editorial boards of several journals, most notably European Journal of Operational Research and Computers and Operations Research. He is the director of the Applied Optimization Systems Group (SOA) at the Instituto Tecnológico de Informática (ITI) where he is or has been the principal investigator in several public research projects as well as privately funded projects with industrial companies. His research interests include scheduling and routing in real life scenarios.
Abstract: Many scheduling problems are simply too hard to be solved exactly, especially for instances of medium or large size. As a result, the literature on heuristics and metaheuristics for scheduling is extensive. Often, metaheuristics can generate solutions close optimality or to tight lower bounds for instances of realistic size in a matter of minutes. Metaheuristics have been refined over the years and there are literally hundreds of papers published every year with applications to most domains in many different journals. Most regrettably, some of these methods are complex in the sense that they have many parameters that affect performance and hence need careful calibration. Furthermore, many times published results are hard to reproduce due to specific speed-ups being used or complicated software constructs. These complex methods are difficult to transfer to industries in the case of scheduling problems. Another important concern is the recently recognized “tsunami” of novel metaheuristics that mimic the most bizarre natural or human processes, as for example intelligent water drops, harmony search, firefly algorithms and the like. See K. Sörensen “Metaheuristics—The Metaphor exposed” (2015), ITOR 22(1):3-18.
In this presentation, we review many different flowshop related problems. From the basic flowshop problem with makespan minimization to other objectives like flowtime minimization, flowshops with sequence-dependent setup times, no-idle flowshops or other variants and extensions, all the way up to complex hybrid flexible flowline problems. We will show how simple Iterated Greedy (IG) algorithms often outperform much more complex approaches. IG methods are inherently simple with very few parameters. They are easy to code and results are easy to reproduce. We will show that for all tested problems so far, they show state-of-the-art performance despite their simplicity. As a result, we will defend the choice of simpler, yet good performing approaches over complicated metaphor-based algorithms.
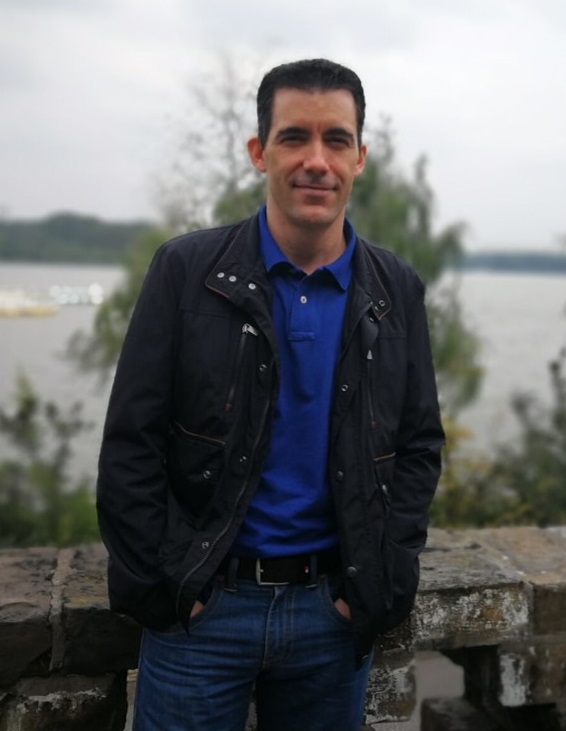